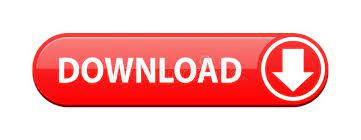
Performing genetic analyses with a sire model gives an estimate of the "sire-variance", which is one fourth of the genetic variance. Thus the sire model is preferred when the model is complex, the amount of data is large, or the analysis has to be repeated many times, as in QTL analyses in which testing many positions is necessary.

The interaction is regarded as significant if the slope variance is significant ].įor the estimation of genotype by environment interactions, both sire models or animal models are used, however sire models are computationally less demanding. sire breeding values regressed on an environmental variable. A common measurement of the interaction is the variance in the slope of the sire reaction norms, i.e. Random regression models are widely used to describe effects that change gradually over a continuous scale, for instance in genotype by environment interaction studies, where the genotype effect is modeled as a function of the environment. Including environmental classes can be used to account for heterogeneous residual variance. When residual variance was heterogeneous, a different approach to account for the heterogeneity of variance was needed, for example when using dairy cattle data in order to prevent overestimation of genetic heterogeneity of variance. This made the ability of sire models to handle heterogeneous genetic variance approximately equivalent to that of animal models. Including an individual effect with a (co)variance matrix restricted to three times the sire (co)variance matrix permitted the modeling of the additive genetic variance not covered by the sire effect. Models differed in their ability to handle heterogeneous variance from different sources. Two animal models were compared to four sire models.
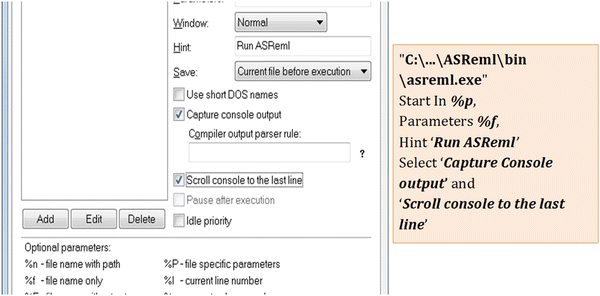
In order to test different models, simulated data with genotype by environment interactions, and dairy cattle data assumed to contain such interactions, were analyzed. The combination of a sire model and a random regression term describing genotype by environment interactions may lead to biased estimates of genetic variance components because of heterogeneous residual variance.
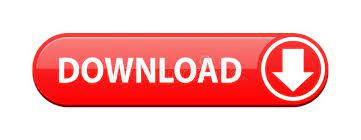